The two worked together to bring a training method called Population Based Training (PBT for short) to bear on Waymo’s challenge of building better virtual drivers, and the results were impressive — DeepMind says in a blog post that using PBT decreased by 24% false positives in a network that identifies and places boxes around pedestrians, bicyclists and motorcyclists spotted by a Waymo vehicle’s many sensors. Not only that, but is also resulted in savings in terms of both training time and resources, using about 50% of both compared to standard methods that Waymo was using previously.
[…]
To step back a little, let’s look at what PBT even is. Basically, it’s a method of training that takes its cues from how Darwinian evolution works. Neural nets essentially work by trying something and then measuring those results against some kind of standard to see if their attempt is more “right” or more “wrong” based on the desired outcome
[…]
But all that comparative training requires a huge amount of resources, and sorting the good from the bad in terms of which are working out relies on either the gut feeling of individual engineers, or massive-scale search with a manual component involved where engineers “weed out” the worst performing neural nets to free up processing capabilities for better ones.
What DeepMind and Waymo did with this experiment was essentially automate that weeding, automatically killing the “bad” training and replacing them with better-performing spin-offs of the best-in-class networks running the task. That’s where evolution comes in, since it’s kind of a process of artificial natural selection.
Wow, I hate when people actually write at you to read a sentence again (cut out for your mental wellness).
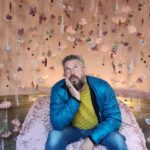
Robin Edgar
Organisational Structures | Technology and Science | Military, IT and Lifestyle consultancy | Social, Broadcast & Cross Media | Flying aircraft