[…] Human brains are slower than machines at processing simple information, such as arithmetic, but they far surpass machines in processing complex information as brains deal better with few and/or uncertain data. Brains can perform both sequential and parallel processing (whereas computers can do only the former), and they outperform computers in decision-making on large, highly heterogeneous, and incomplete datasets and other challenging forms of processing
[…]
fundamental differences between biological and machine learning in the mechanisms of implementation and their goals result in two drastically different efficiencies. First, biological learning uses far less power to solve computational problems. For example, a larval zebrafish navigates the world to successfully hunt prey and avoid predators (4) using only 0.1 microwatts (5), while a human adult consumes 100 watts, of which brain consumption constitutes 20% (6, 7). In contrast, clusters used to master state-of-the-art machine learning models typically operate at around 106 watts.
[…]
biological learning uses fewer observations to learn how to solve problems. For example, humans learn a simple “same-versus-different” task using around 10 training samples (12); simpler organisms, such as honeybees, also need remarkably few samples (~102) (13). In contrast, in 2011, machines could not learn these distinctions even with 106 samples (14) and in 2018, 107 samples remained insufficient (15). Thus, in this sense, at least, humans operate at a >106 times better data efficiency than modern machines
[…]
The power and efficiency advantages of biological computing over machine learning are multiplicative. If it takes the same amount of time per sample in a human or machine, then the total energy spent to learn a new task requires 1010 times more energy for the machine.
[…]
We have coined the term “organoid intelligence” (OI) to describe an emerging field aiming to expand the definition of biocomputing toward brain-directed OI computing, i.e. to leverage the self-assembled machinery of 3D human brain cell cultures (brain organoids) to memorize and compute inputs.
[…]
In this article, we present an architecture (Figure 1) and blueprint for an OI development and implementation program designed to:
● Determine the biofeedback characteristics of existing human brain organoids caged in microelectrode shells, potentially using AI to analyze recorded response patterns to electrical and chemical (neurotransmitters and their corresponding receptor agonists and antagonists) stimuli.
● Empirically test, refine, and, where needed, develop neurocomputational theories that elucidate the basis of in vivo biological intelligence and allow us to interact with and harness an OI system.
● Further scale up the brain organoid model to increase the quantity of biological matter, the complexity of brain organoids, the number of electrodes, algorithms for real-time interactions with brain organoids, and the connected input sources and output devices; and to develop big-data warehousing and machine learning methods to accommodate the resulting brain-directed computing capacity.
● Explore how this program could improve our understanding of the pathophysiology of neurodevelopmental and neurodegenerative disorders toward innovative approaches to treatment or prevention.
● Establish a community and a large-scale project to realize OI computing, taking full account of its ethical implications and developing a common ontology.
FIGURE 1Figure 1 Architecture of an OI system for biological computing. At the core of OI is the 3D brain cell culture (organoid) that performs the computation. The learning potential of the organoid is optimized by culture conditions and enrichment by cells and genes critical for learning (including IEGs). The scalability, viability, and durability of the organoid are supported by integrated microfluidic systems. Various types of input can be provided to the organoid, including electrical and chemical signals, synthetic signals from machine sensors, and natural signals from connected sensory organoids (e.g. retinal). We anticipate high-resolution output measurement both by electrophysiological recordings obtained via specially designed 2D or 3D (shell) MEA, and potentially from implantable probes, and imaging of organoid structural and functional properties. These outputs can be used directly for computation purposes and as biofeedback to promote organoid learning. AI and machine learning are used throughout to encode and decode signals and to develop hybrid biocomputing solutions, in conjunction with a suitable big-data management system.
To the latter point, a community-forming workshop was held in February 2022 (51), which gave rise to the Baltimore Declaration Toward OI (52). It provides a statement of vision for an OI community that has led to the development of the program outlined here.
[…]
The past decade has seen a revolution in brain cell cultures, moving from traditional monolayer cultures to more organ-like, organized 3D cultures – i.e. brain organoids (Figure 2A). These can be generated either from embryonic stem cells or from the less ethically problematic iPSC typically derived from skin samples (54). The Johns Hopkins Center for Alternatives to Animal Testing, among others, has produced such brain organoids with high levels of standardization and scalability (32) (Figure 2B). Having a diameter below 500 μm, and comprising fewer than 100,000 cells, each organoid is roughly one 3-millionth the size of the human brain (theoretically equating to 800 MB of memory storage). Other groups have reported brain organoids with average diameters of 3–5 mm and prolonged culture times exceeding 1 year (34–36, 55–59).
FIGURE 2Figure 2 Advances in 3D cell culturing provide the foundation for systems to explore organoid intelligence. (A) 3D neural cell cultures have important advantages for biological learning, compared with conventional 2D monolayers – namely a far greater density of cells, enhanced synaptogenesis, high levels of myelination, and enrichment by cell types essential to learning. (B) Brain organoid differentiation over time from 4 to 15 weeks, showing neurons (microtubule associated protein 2 [MAP2]; pink), oligodendrocytes (oligodendrocyte transcription factor [OLIG2]; red), and astrocytes (glial fibrillary acidic protein [GFAP]; green). Nuclei are stained with Hoechst 33342 (blue). Images were taken with an LCM 880 confocal microscope with 20x and 63x magnification. Scale bars are 100 μm and 20 μm, respectively. The images show the presence of MAP2-positive neurons as early as 4 weeks, while glial cells emerge at 8 weeks and there is a continuous increase in the number of astrocytes over time.
These organoids show various attributes that should improve their potential for biocomputing (Figure 2).
[…]
axons in these organoids show extensive myelination. Pamies et al. were the first to develop a 3D human brain model showing significant myelination of axons (32). About 40% of axons in the brain organoids were myelinated (30, 31), which approaches the 50% found in the human brain (60, 61). Myelination has since been reproduced in other brain organoids (47, 62). Myelin reduces the capacitance of the axonal membrane and enables saltatory conduction from one node of Ranvier to the next. As myelination increases electrical conductivity approximately 100-fold, this promises to boost biological computing performance, though its functional impact in this model remains to be demonstrated.
Finally, these organoid cultures can be enriched with various cell types involved in biological learning, namely oligodendrocytes, microglia, and astrocytes. Glia cells are integrally important for the pruning of synapses in biological learning (63–65) but have not yet been reported at physiologically relevant levels in brain organoid models. Preliminary work in our organoid model has shown the potential for astroglia cell expansion to physiologically relevant levels (47). Furthermore, recent evidence that oligodendrocytes and astrocytes significantly contribute to learning plasticity and memory suggests that these processes should be studied from a neuron-to-glia perspective, rather than the neuron-to-neuron paradigm generally used (63–65). In addition, optimizing the cell culture conditions to allow the expression of immediate early genes (IEGs) is expected to further boost the learning and memory capacities of brain organoids since these are key to learning processes and are expressed only in neurons involved in memory formation
[…]
Source: Frontiers | Organoid intelligence (OI): the new frontier in biocomputing and intelligence-in-a-dish
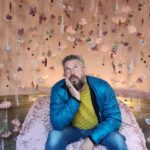
Robin Edgar
Organisational Structures | Technology and Science | Military, IT and Lifestyle consultancy | Social, Broadcast & Cross Media | Flying aircraft