Most recent advances in artificial-intelligence systems such as speech- or face-recognition programs have come courtesy of neural networks, densely interconnected meshes of simple information processors that learn to perform tasks by analyzing huge sets of training data.
But neural nets are large, and their computations are energy intensive, so they’re not very practical for handheld devices. Most smartphone apps that rely on neural nets simply upload data to internet servers, which process it and send the results back to the phone.
Now, MIT researchers have developed a special-purpose chip that increases the speed of neural-network computations by three to seven times over its predecessors, while reducing power consumption 94 to 95 percent. That could make it practical to run neural networks locally on smartphones or even to embed them in household appliances.
“The general processor model is that there is a memory in some part of the chip, and there is a processor in another part of the chip, and you move the data back and forth between them when you do these computations,” says Avishek Biswas, an MIT graduate student in electrical engineering and computer science, who led the new chip’s development.
“Since these machine-learning algorithms need so many computations, this transferring back and forth of data is the dominant portion of the energy consumption. But the computation these algorithms do can be simplified to one specific operation, called the dot product. Our approach was, can we implement this dot-product functionality inside the memory so that you don’t need to transfer this data back and forth?”
Source: Neural networks everywhere | MIT News
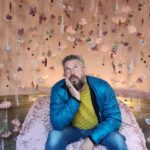
Robin Edgar
Organisational Structures | Technology and Science | Military, IT and Lifestyle consultancy | Social, Broadcast & Cross Media | Flying aircraft