On Monday, a group of AI researchers from Google, DeepMind, UC Berkeley, Princeton, and ETH Zurich released a paper outlining an adversarial attack that can extract a small percentage of training images from latent diffusion AI image synthesis models like Stable Diffusion. It challenges views that image synthesis models do not memorize their training data and that training data might remain private if not disclosed. Recently, AI image synthesis models have been the subject of intense ethical debate and even legal action. Proponents and opponents of generative AI tools regularly argue over the privacy and copyright implications of these new technologies. Adding fuel to either side of the argument could dramatically affect potential legal regulation of the technology, and as a result, this latest paper, authored by Nicholas Carlini et al., has perked up ears in AI circles.
However, Carlini’s results are not as clear-cut as they may first appear. Discovering instances of memorization in Stable Diffusion required 175 million image generations for testing and preexisting knowledge of trained images. Researchers only extracted 94 direct matches and 109 perceptual near-matches out of 350,000 high-probability-of-memorization images they tested (a set of known duplicates in the 160 million-image dataset used to train Stable Diffusion), resulting in a roughly 0.03 percent memorization rate in this particular scenario. Also, the researchers note that the “memorization” they’ve discovered is approximate since the AI model cannot produce identical byte-for-byte copies of the training images. By definition, Stable Diffusion cannot memorize large amounts of data because the size of the 160,000 million-image training dataset is many orders of magnitude larger than the 2GB Stable Diffusion AI model. That means any memorization that exists in the model is small, rare, and very difficult to accidentally extract.
Still, even when present in very small quantities, the paper appears to show that approximate memorization in latent diffusion models does exist, and that could have implications for data privacy and copyright. The results may one day affect potential image synthesis regulation if the AI models become considered “lossy databases” that can reproduce training data, as one AI pundit speculated. Although considering the 0.03 percent hit rate, they would have to be considered very, very lossy databases — perhaps to a statistically insignificant degree. […] Eric Wallace, one of the paper’s authors, shared some personal thoughts on the research in a Twitter thread. As stated in the paper, he suggested that AI model-makers should de-duplicate their data to reduce memorization. He also noted that Stable Diffusion’s model is small relative to its training set, so larger diffusion models are likely to memorize more. And he advised against applying today’s diffusion models to privacy-sensitive domains like medical imagery.
Source: Stable Diffusion ‘Memorizes’ Some Images, Sparking Privacy Concerns – Slashdot
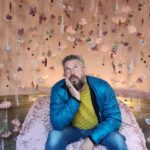
Robin Edgar
Organisational Structures | Technology and Science | Military, IT and Lifestyle consultancy | Social, Broadcast & Cross Media | Flying aircraft