[…]
One of the first, and reportedly most widely used, is PredPol, its name an amalgamation of the words “predictive policing.” The software was derived from an algorithm used to predict earthquake aftershocks that was developed by professors at UCLA and released in 2011. By sending officers to patrol these algorithmically predicted hot spots, these programs promise they will deter illegal behavior.
But law enforcement critics had their own prediction: that the algorithms would send cops to patrol the same neighborhoods they say police always have, those populated by people of color. Because the software relies on past crime data, they said, it would reproduce police departments’ ingrained patterns and perpetuate racial injustice, covering it with a veneer of objective, data-driven science.
PredPol has repeatedly said those criticisms are off-base. The algorithm doesn’t incorporate race data, which, the company says, “eliminates the possibility for privacy or civil rights violations seen with other intelligence-led or predictive policing models.”
There have been few independent, empirical reviews of predictive policing software because the companies that make these programs have not publicly released their raw data.
A seminal, data-driven study about PredPol published in 2016 did not involve actual predictions. Rather the researchers, Kristian Lum and William Isaac, fed drug crime data from Oakland, California, into PredPol’s open-source algorithm to see what it would predict. They found that it would have disproportionately targeted Black and Latino neighborhoods, despite survey data that shows people of all races use drugs at similar rates.
PredPol’s founders conducted their own research two years later using Los Angeles data and said they found the overall rate of arrests for people of color was about the same whether PredPol software or human police analysts made the crime hot spot predictions. Their point was that their software was not worse in terms of arrests for people of color than nonalgorithmic policing.
However, a study published in 2018 by a team of researchers led by one of PredPol’s founders showed that Indianapolis’s Latino population would have endured “from 200% to 400% the amount of patrol as white populations” had it been deployed there, and its Black population would have been subjected to “150% to 250% the amount of patrol compared to white populations.” The researchers said they found a way to tweak the algorithm to reduce that disproportion but that it would result in less accurate predictions—though they said it would still be “potentially more accurate” than human predictions.
[…]
Other predictive police programs have also come under scrutiny. In 2017, the Chicago Sun-Times obtained a database of the city’s Strategic Subject List, which used an algorithm to identify people at risk of becoming victims or perpetrators of violent, gun-related crime. The newspaper reported that 85% of people that the algorithm saddled with the highest risk scores were Black men—some with no violent criminal record whatsoever.
Last year, the Tampa Bay Times published an investigation analyzing the list of people that were forecast to commit future crimes by the Pasco Sheriff’s Office’s predictive tools. Deputies were dispatched to check on people on the list more than 12,500 times. The newspaper reported that at least one in 10 of the people on the list were minors, and many of those young people had only one or two prior arrests yet were subjected to thousands of checks.
For our analysis, we obtained a trove of PredPol crime prediction data that has never before been released by PredPol for unaffiliated academic or journalistic analysis. Gizmodo found it exposed on the open web (the portal is now secured) and downloaded more than 7 million PredPol crime predictions for dozens of American cities and some overseas locations between 2018 and 2021.
[…]
rom Fresno, California, to Niles, Illinois, to Orange County, Florida, to Piscataway, New Jersey. We supplemented our inquiry with Census data, including racial and ethnic identities and household incomes of people living in each jurisdiction—both in areas that the algorithm targeted for enforcement and those it did not target.
Overall, we found that PredPol’s algorithm relentlessly targeted the Census block groups in each jurisdiction that were the most heavily populated by people of color and the poor, particularly those containing public and subsidized housing. The algorithm generated far fewer predictions for block groups with more White residents.
Analyzing entire jurisdictions, we observed that the proportion of Black and Latino residents was higher in the most-targeted block groups and lower in the least-targeted block groups (about 10% of which had zero predictions) compared to the overall jurisdiction. We also observed the opposite trend for the White population: The least-targeted block groups contained a higher proportion of White residents than the jurisdiction overall, and the most-targeted block groups contained a lower proportion.
[…]
We also found that PredPol’s predictions often fell disproportionately in places where the poorest residents live
[…]
To try to determine the effects of PredPol predictions on crime and policing, we filed more than 100 public records requests and compiled a database of more than 600,000 arrests, police stops, and use-of-force incidents. But most agencies refused to give us any data. Only 11 provided at least some of the necessary data.
For the 11 departments that provided arrest data, we found that rates of arrest in predicted areas remained the same whether PredPol predicted a crime that day or not. In other words, we did not find a strong correlation between arrests and predictions. (See the Limitations section for more information about this analysis.)
We do not definitively know how police acted on any individual crime prediction because we were refused that data by nearly every police department.
[…]
Overall, our analysis suggests that the algorithm, at best, reproduced how officers have been policing, and at worst, would reinforce those patterns if its policing recommendations were followed.
[…]
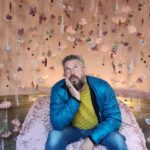
Robin Edgar
Organisational Structures | Technology and Science | Military, IT and Lifestyle consultancy | Social, Broadcast & Cross Media | Flying aircraft