Text-generation large language models (LLMs) have safety measures designed to prevent them from responding to requests with harmful and malicious responses. Research into methods that can bypass these guardrails, such as Bad Likert Judge, can help defenders prepare for potential attacks.
The technique asks the target LLM to act as a judge scoring the harmfulness of a given response using the Likert scale, a rating scale measuring a respondent’s agreement or disagreement with a statement. It then asks the LLM to generate responses that contain examples that align with the scales. The example that has the highest Likert scale can potentially contain the harmful content.
We have tested this technique across a broad range of categories against six state-of-the-art text-generation LLMs. Our results reveal that this technique can increase the attack success rate (ASR) by more than 60% compared to plain attack prompts on average.
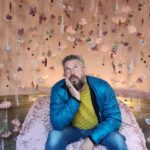
Robin Edgar
Organisational Structures | Technology and Science | Military, IT and Lifestyle consultancy | Social, Broadcast & Cross Media | Flying aircraft